Full Publication List

Grok, our most advanced and truth-seeking model: blending strong reasoning with extensive pretraining knowledge.
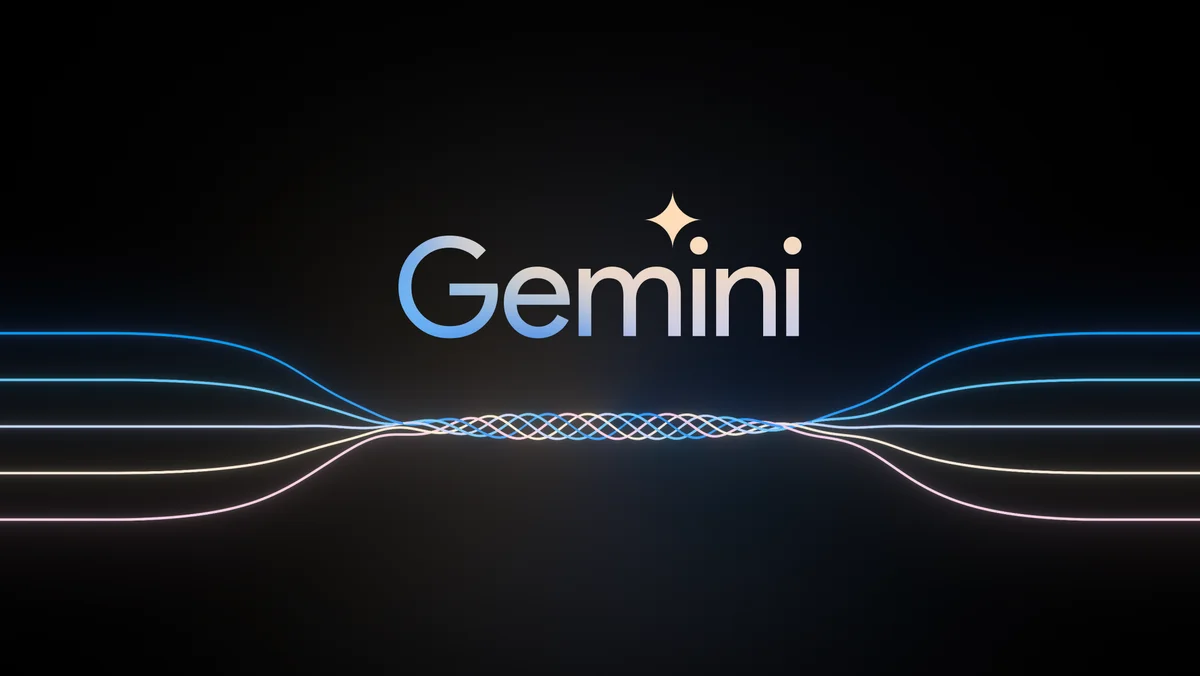
This paper presents Instruct-Imagen, a model that tackles heterogeneous image generation tasks and generalizes across unseen tasks.
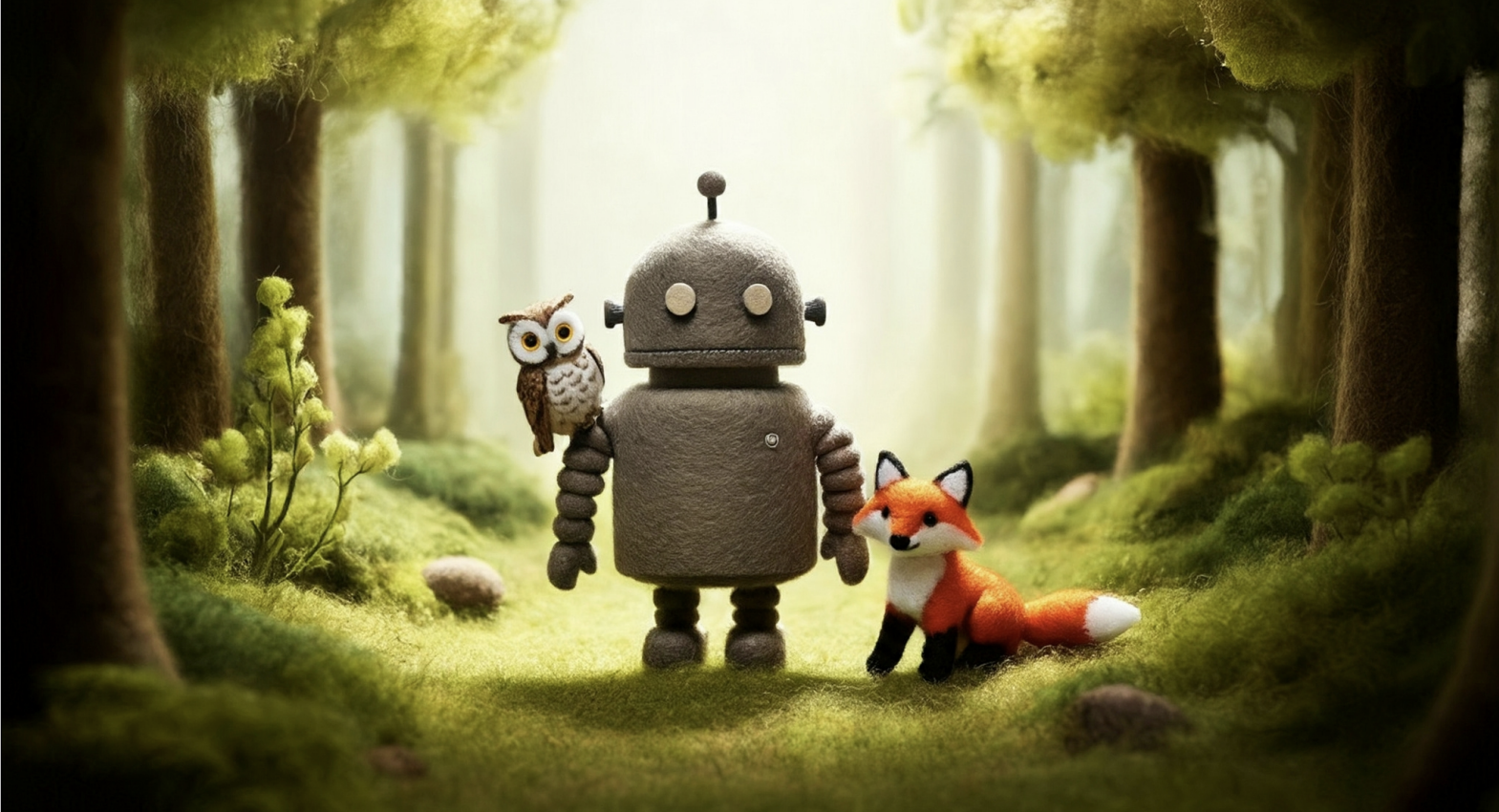
We introduce Imagen 3, a latent diffusion model that generates high quality images from text prompts. We describe our quality and responsibility evaluations. Imagen 3 is preferred over other state-of-the-art (SOTA) models at the time of evaluation. In addition, we discuss issues around safety and representation, as well as methods we used to minimize the potential harm of our models.
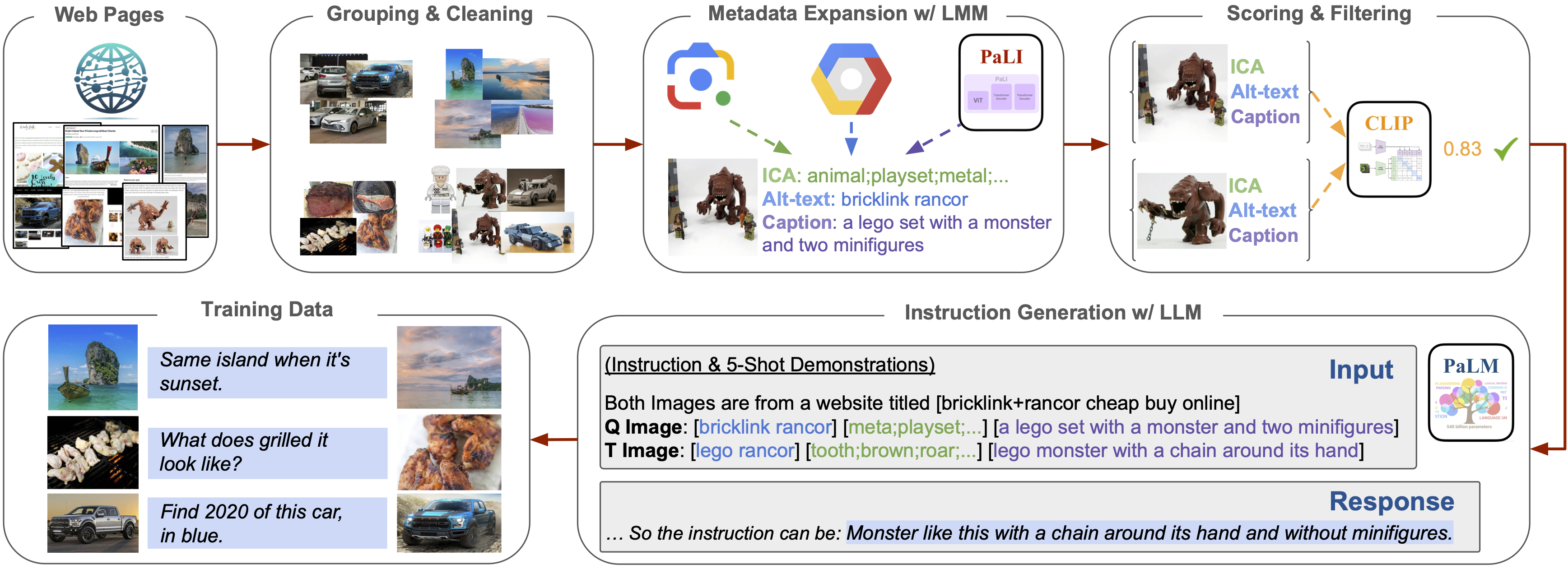
We introduce MagicLens, a series of self-supervised image retrieval models that support open-ended instructions. MagicLens is built on a key novel insight: image pairs that naturally occur on the same web pages contain a wide range of implicit relations (e.g., inside view of), and we can bring those implicit relations explicit by synthesizing instructions via large multimodal models (LMMs) and large language models (LLMs). Trained on 36.7M (query image, instruction, target image) triplets with rich semantic relations mined from the web, MagicLens achieves comparable or better results on eight benchmarks of various image retrieval tasks than prior state-of-the-art (SOTA) methods. Remarkably, it outperforms previous SOTA but with a 50X smaller model size on multiple benchmarks. Additional human analyses on a 1.4M-image unseen corpus further demonstrate the diversity of search intents supported by MagicLens.
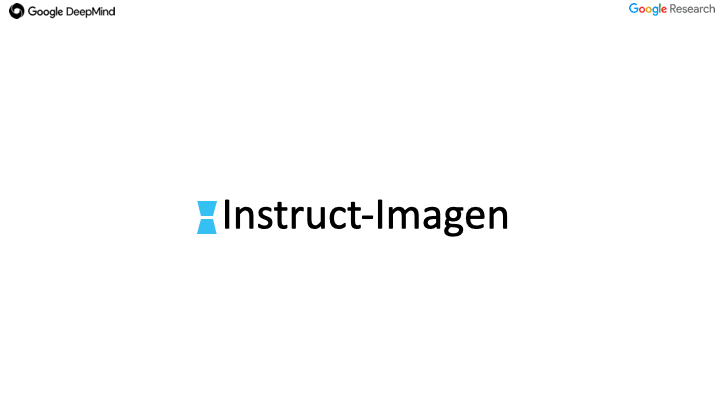
This paper presents Instruct-Imagen, a model that tackles heterogeneous image generation tasks and generalizes across unseen tasks.
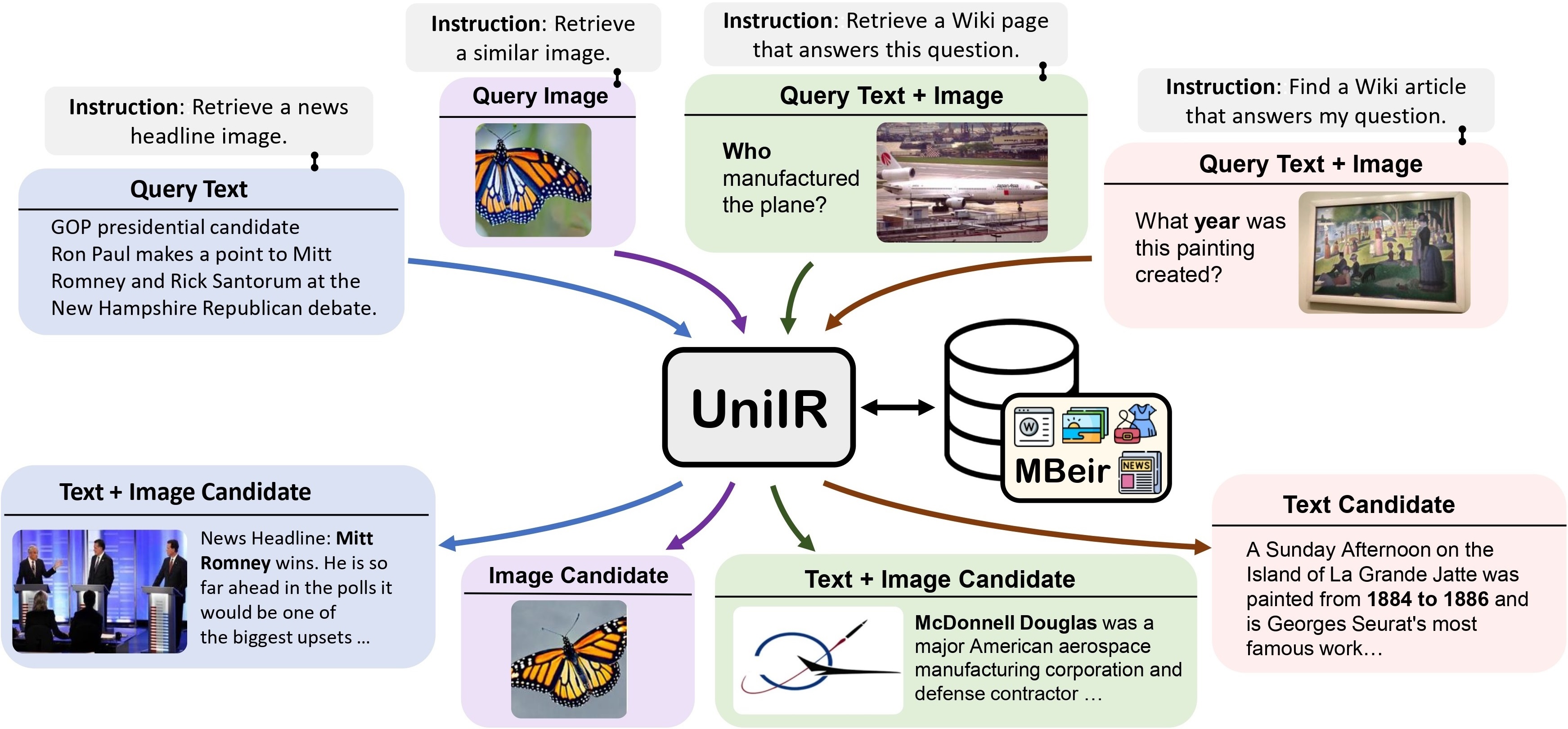
We introduce UniIR, a unified instruction-guided multimodal retriever capable of handling eight distinct retrieval tasks across modalities.
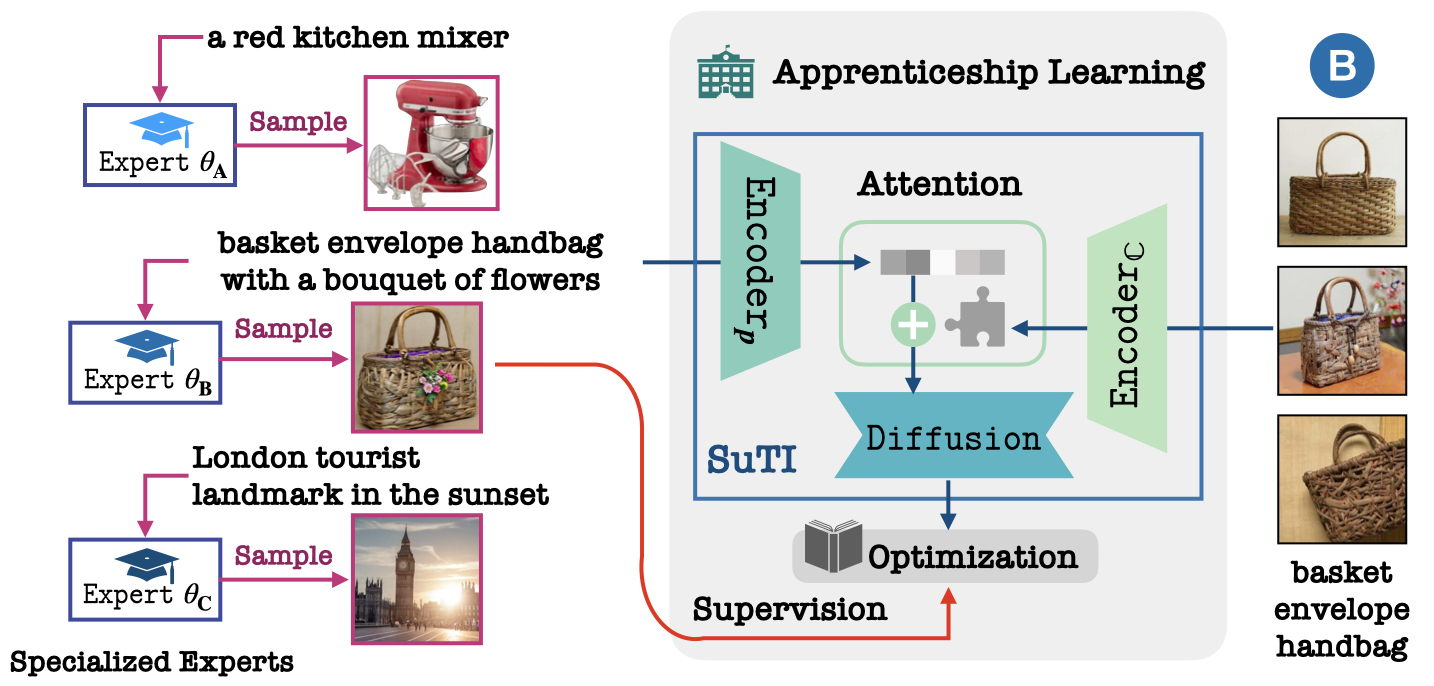
We present SuTI, a Subject-driven Text-to-Image generator that replaces subject-specific fine tuning with in-context learning. Given a few demonstrations of a new subject, SuTI can instantly generate novel renditions of the subject in different scenes, without any subject-specific optimization. SuTI is powered by apprenticeship learning, where a single apprentice model is learned from data generated by massive amount of subject-specific expert models.
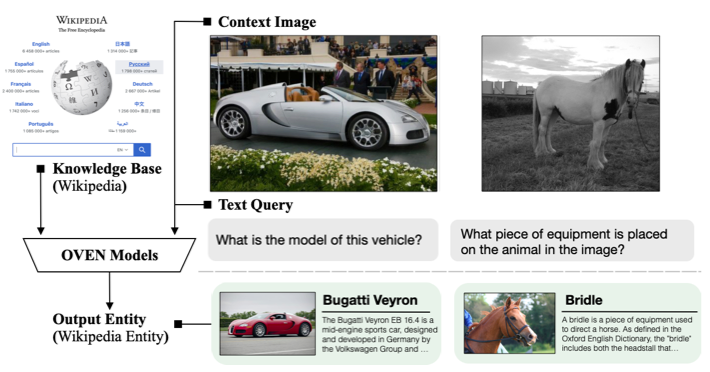
Large-scale multi-modal pre-training models such as CLIP and PaLI exhibit strong generalization on various visual domains and tasks. However, existing image classification benchmarks often evaluate recognition on a specific domain (e.g., outdoor images) or a specific task (e.g., classifying plant species), which falls short of evaluating whether pre-trained foundational models are universal visual recognizers. To address this, we formally present the task of Open-domain Visual Entity recognitioN (OVEN), where a model need to link an image onto a Wikipedia entity with respect to a text query. We construct OVEN-Wiki by re-purposing 14 existing datasets with all labels grounded onto one single label space: Wikipedia entities. OVEN challenges models to select among six million possible Wikipedia entities, making it a general visual recognition benchmark with the largest number of labels.
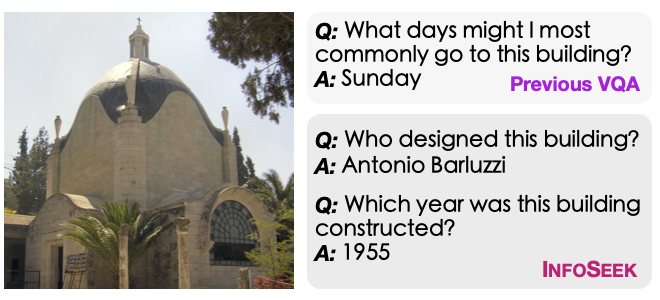
Can pre-trained vision and langauge models understand how to answer visual information seeking questions? To answer this question, we present InfoSeek, a Visual Question Answering dataset that focuses on asking information-seeking questions, where the information can not be answered by common sense knowledge. We perform a multi-stage human annotation to collect a natural distribution of high-quality visual information seeking question-answer pairs. We also construct a large-scale, automatically collected dataset by combining existing visual entity recognition datasets and Wikidata, which provides over one million examples for model fine-tuning and validation.
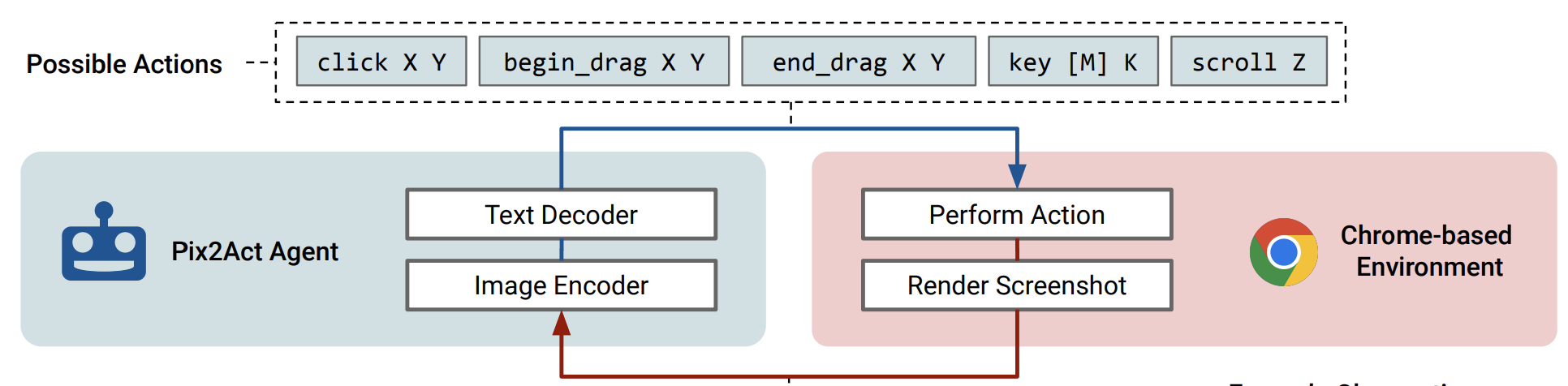
This paper focuses on creating agents that interact with the digital world using the same conceptual interface that humans commonly use — via pixel-based screenshots and a generic action space corresponding to keyboard and mouse actions. Building upon recent progress in pixel-based pretraining, we show, for the first time, that it is possible for such agents to outperform human crowdworkers on the MiniWob++ benchmark of GUI-based instruction following tasks.
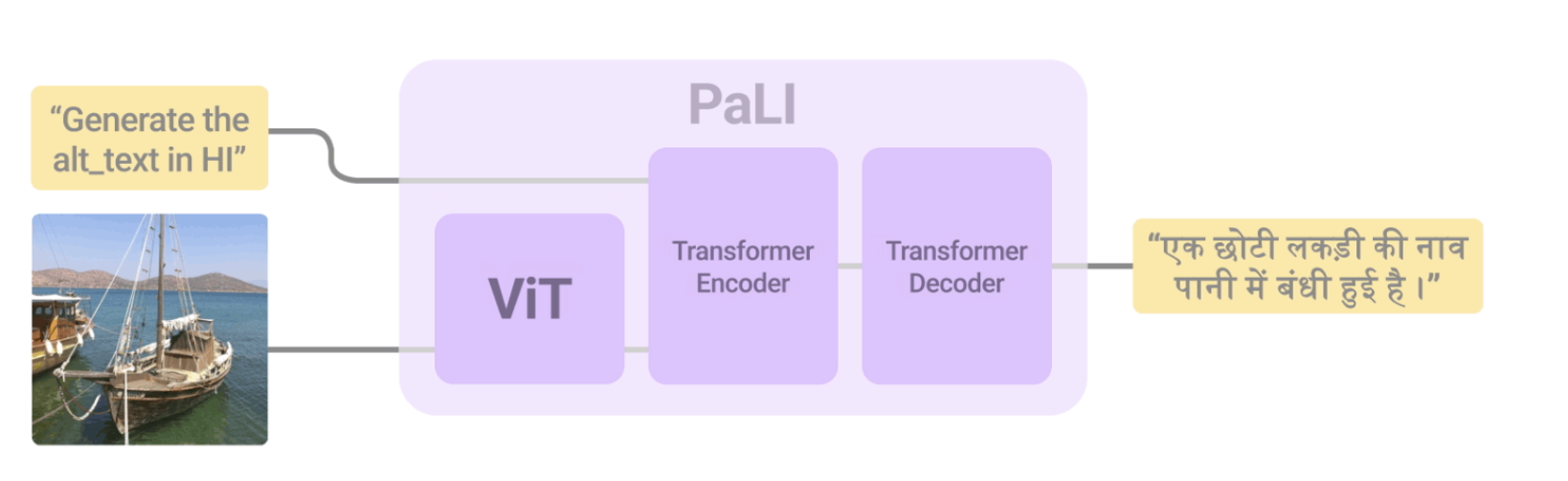
We present the training recipe and results of scaling up PaLI-X, a multilingual vision and language model, both in terms of size of the components and the breadth of its training task mixture. Our model achieves new levels of performance on a wide-range of varied and complex tasks, including multiple image-based captioning and question-answering tasks, image-based document understanding and few-shot (in-context) learning, as well as object detection, video question answering, and video captioning.
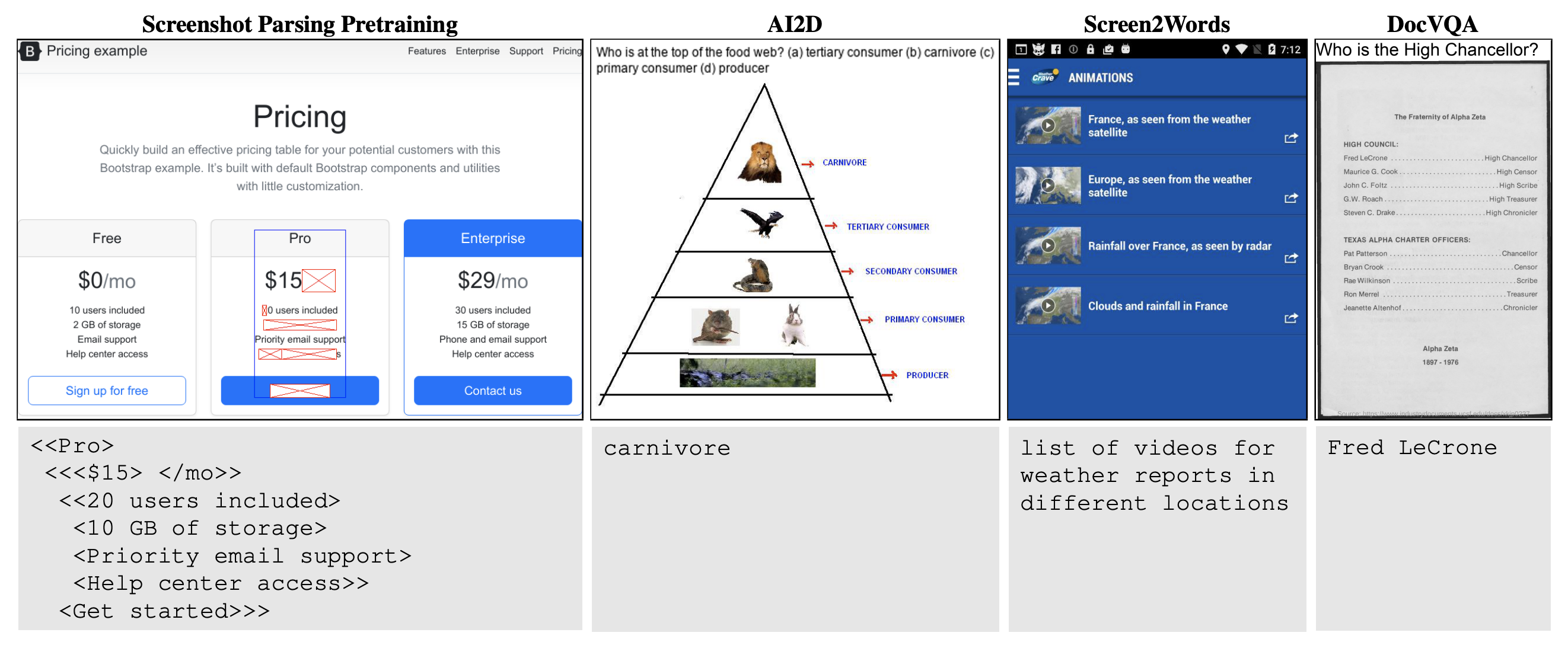
We present Pix2Struct, a pretrained image-to-text model for purely visual language understanding, which can be finetuned on tasks containing visually-situated language. Pix2Struct is pretrained by learning to parse masked screenshots of web pages into simplified HTML.
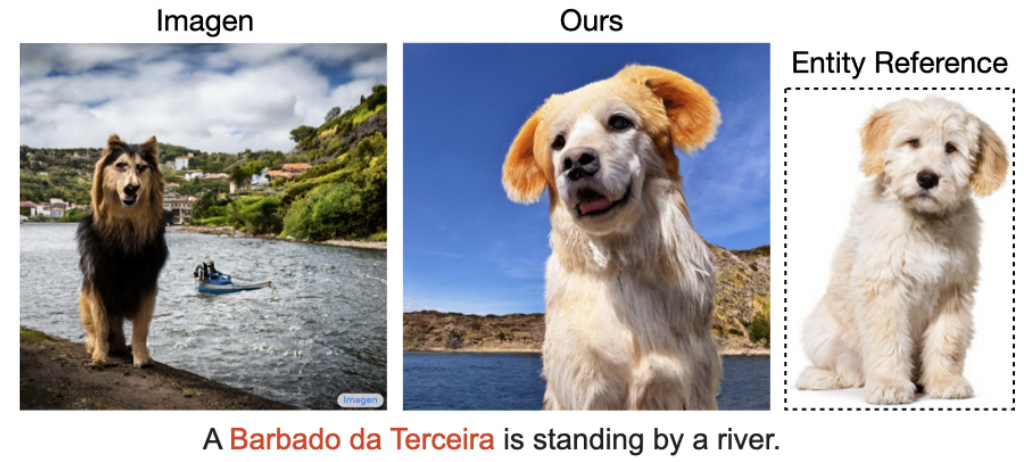
We present the Retrieval-Augmented Text-to-Image Generator (Re-Imagen), a generative model that uses retrieved information to produce high-fidelity and faithful images, even for rare or unseen entities. Given a text prompt, Re-Imagen accesses an external multi-modal knowledge base to retrieve relevant (image, text) pairs, and uses them as references to generate the image. With this retrieval step, Re-Imagen is augmented with the knowledge of high-level semantics and low-level visual details of the mentioned entities, and thus improves its accuracy in generating the entities' visual appearances.
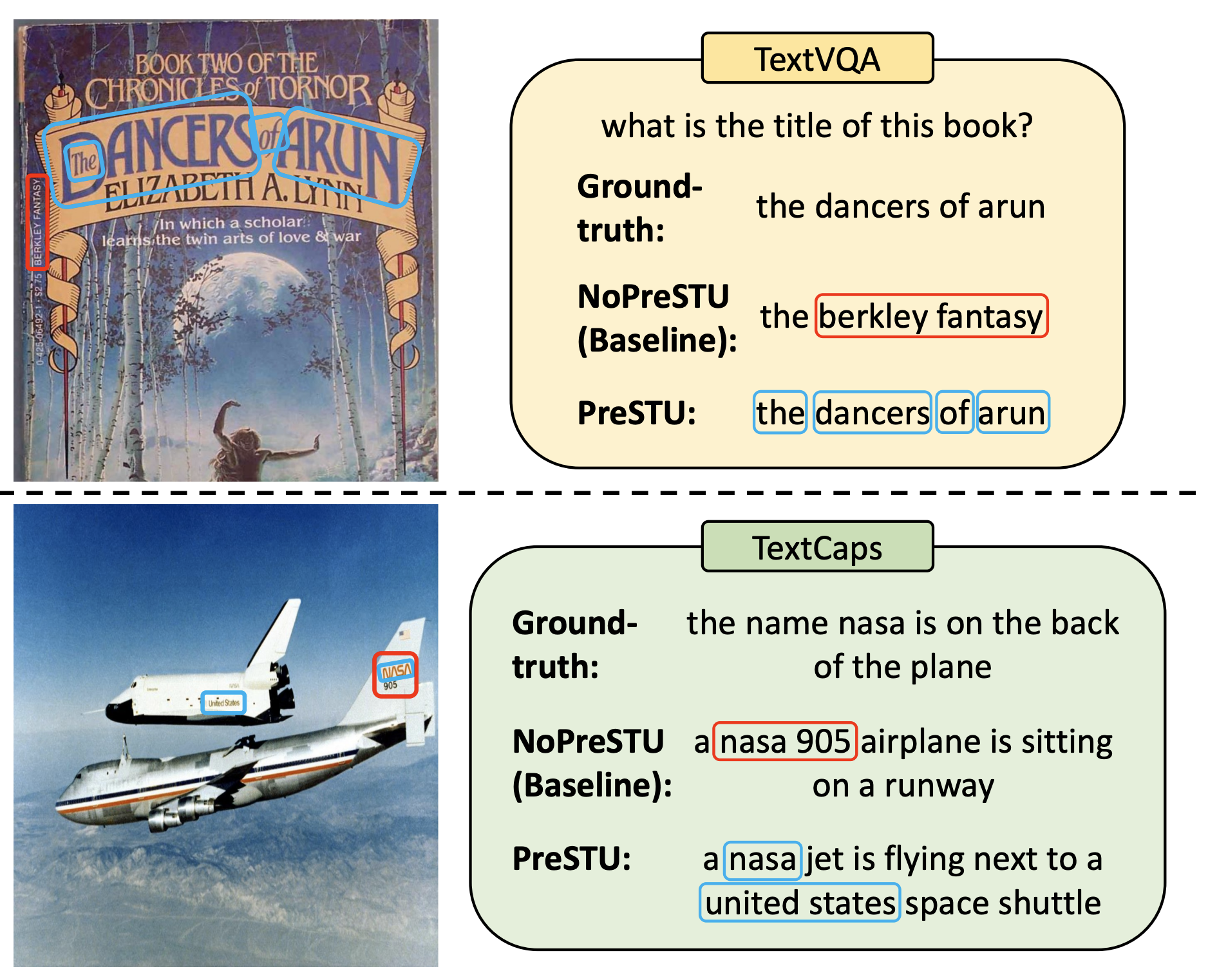
In this paper, we propose PreSTU, a simple pre-training recipe specifically designed for scene-text understanding. PreSTU combines a simple OCR-aware pre-training objective with a large-scale image-text dataset with off-the-shelf OCR signals.
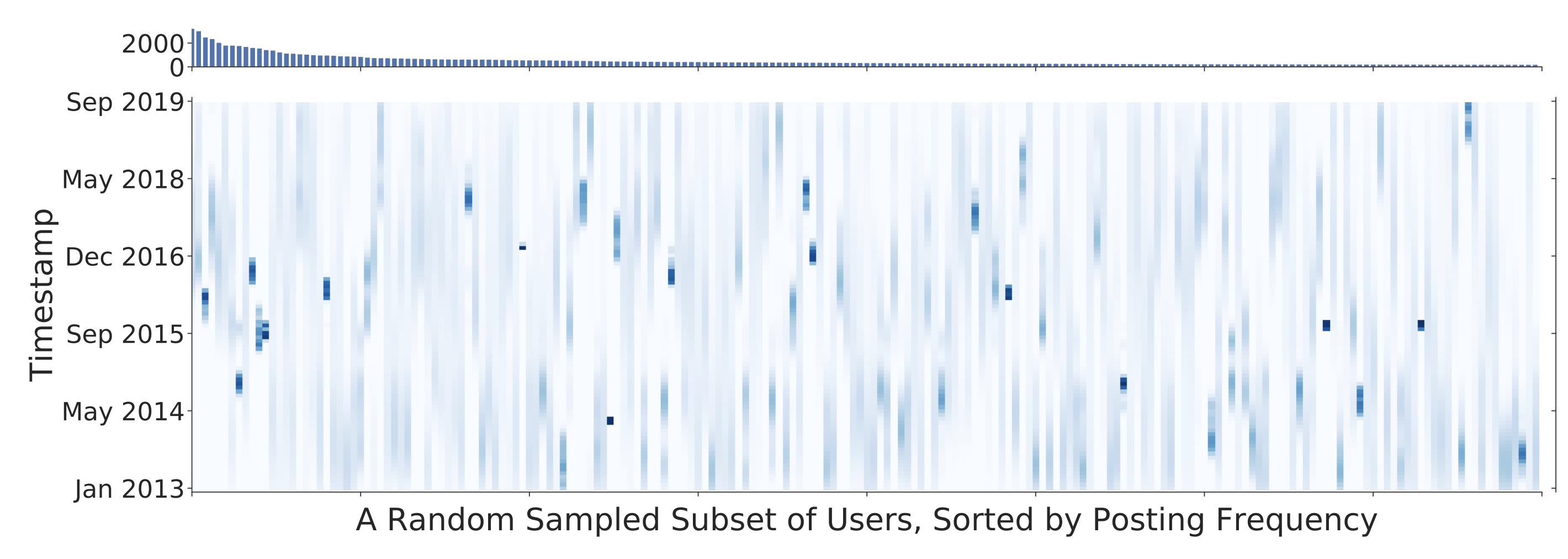
We study a natural setting for continual learning on a massive scale. We introduce the problem of personalized online language learning (POLL), which involves fitting personalized language models to a population of users that evolves over time. To facilitate research on POLL, we collect massive datasets of Twitter posts. These datasets, Firehose10M and Firehose100M, comprise 100 million tweets, posted by one million users over six years.
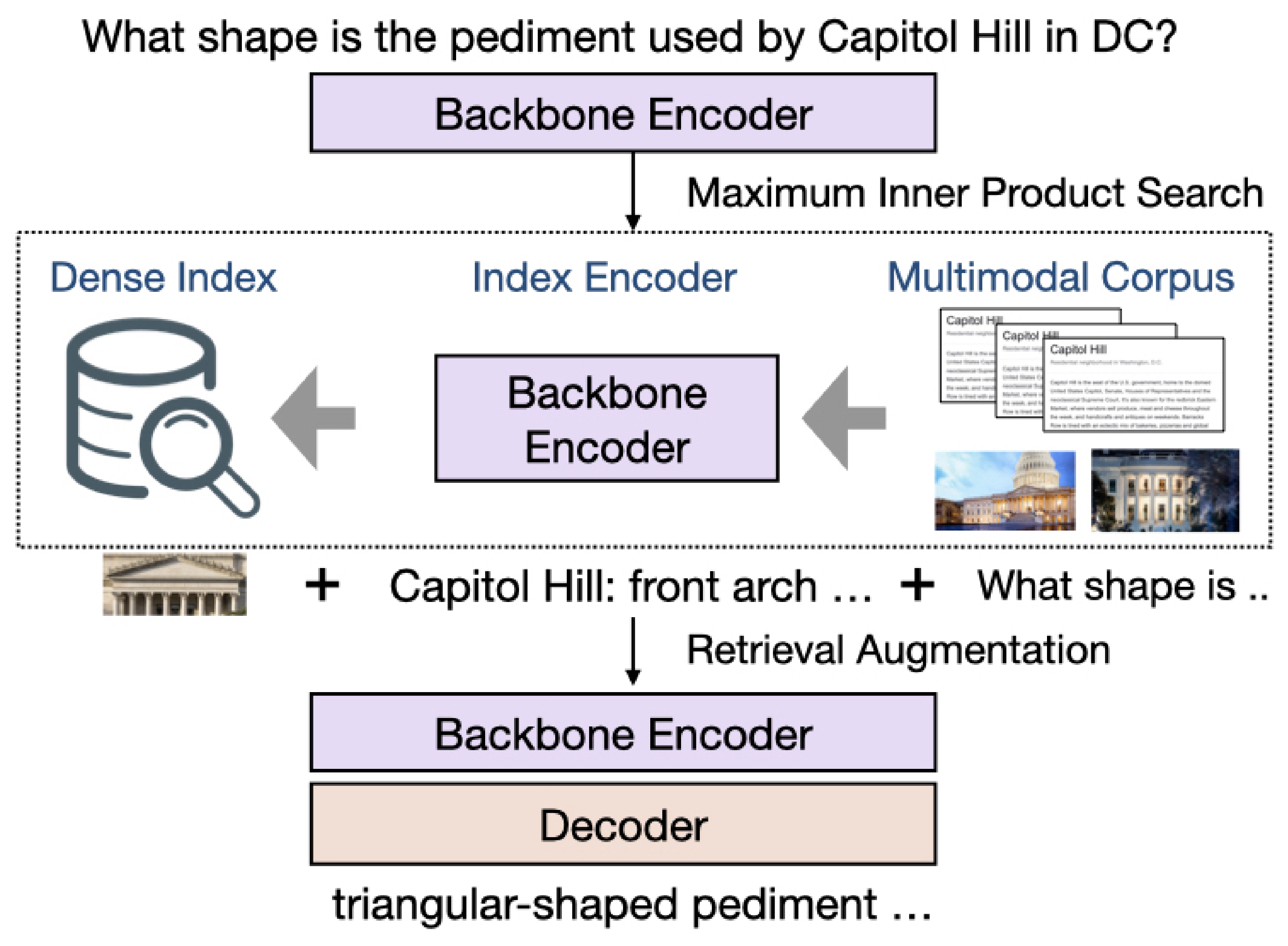
We propose the first Multimodal Retrieval-Augmented Transformer (MuRAG), which accesses an external non-parametric multimodal memory to augment language generation. MuRAG is pre-trained with a mixture of large-scale image-text and text-only corpora using a joint contrastive and generative loss.
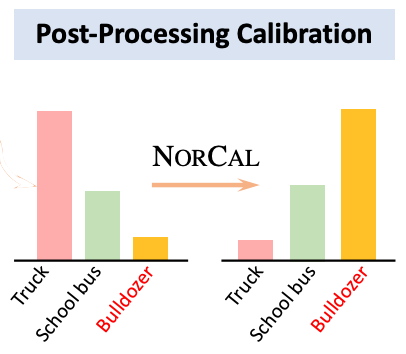
We propose NorCal, Normalized Calibration for long-tailed object detection and instance segmentation, a simple and straightforward recipe that reweighs the predicted scores of each class by its training sample size. We show that separately handling the background class and normalizing the scores over classes for each proposal are keys to achieving superior performance.
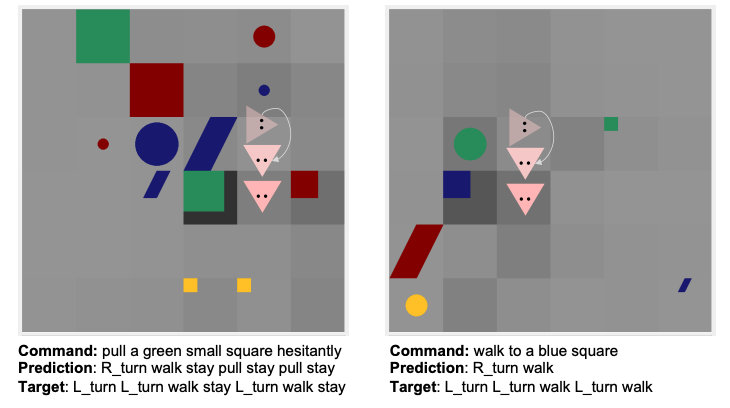
We analyze the grounded SCAN (gSCAN) benchmark, which was recently proposed to study systematic generalization for grounded language understanding.
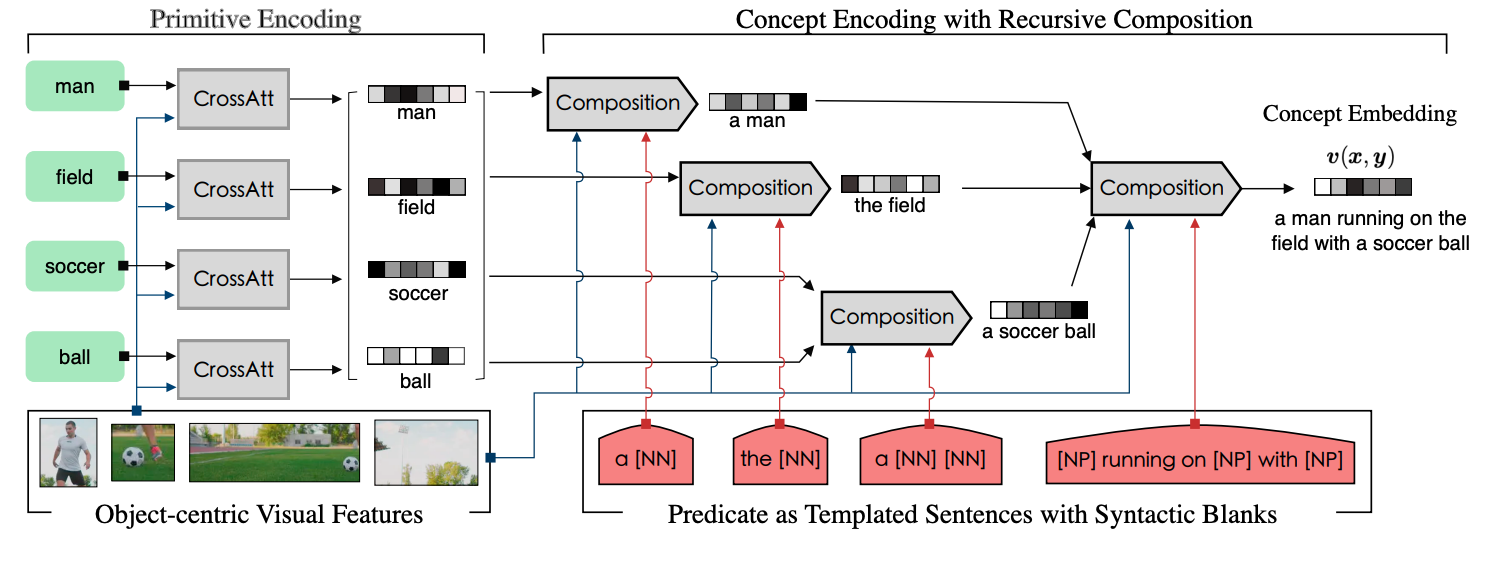
We investigate ways to compose complex concepts in texts from primitive ones while grounding them in images. We propose Concept and Relation Graph (CRG), which builds on top of constituency analysis and consists of recursively combined concepts with predicate functions.
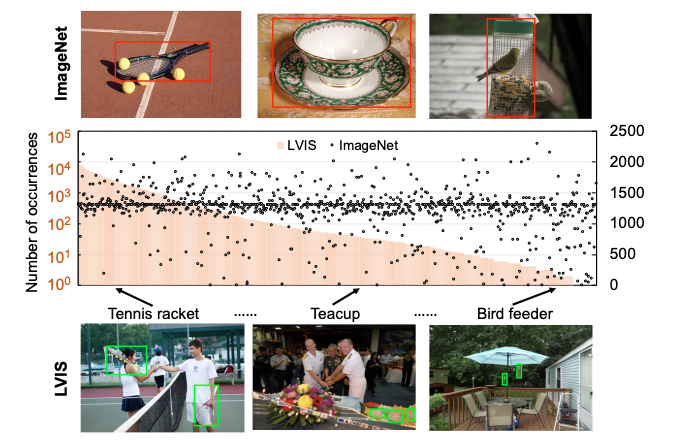
We propose Mosaic of Object-centric images as Scene-centric images (MosaicOS), a simple and novel framework that is surprisingly effective at tackling the challenges of long-tailed object detection.
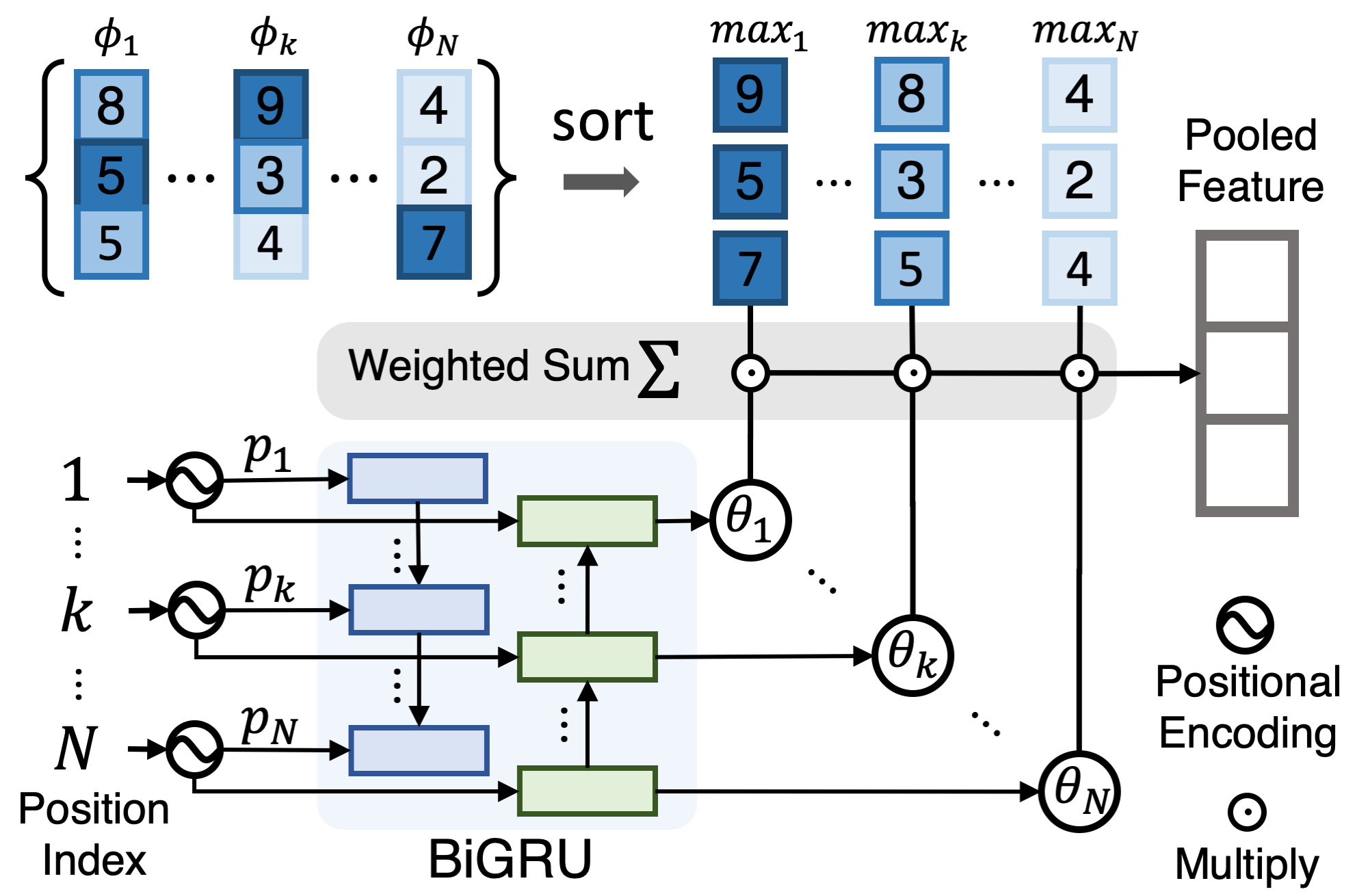
We propose a Generalized Pooling Operator (GPO), which learns to automatically adapt itself to the best pooling strategy for different feature modalities in visual semantic embedding models, requiring no manual tuning while staying effective and efficient.
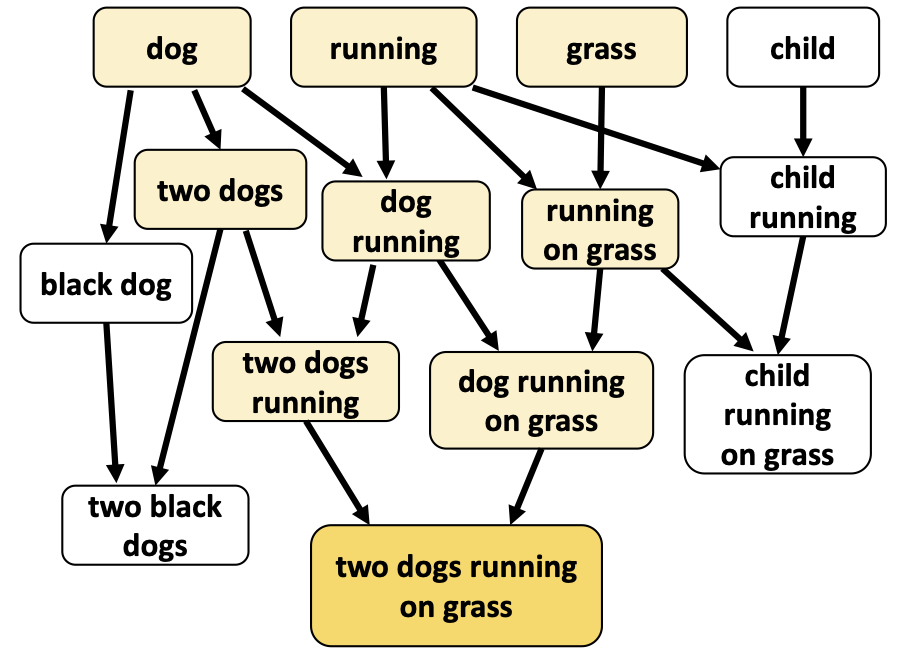
In this paper, we propose learning representations from a set of implied, visually grounded expressions between image and text, automatically mined from those datasets. In particular, we use denotation graphs to represent how specific concepts (such as sentences describing images) can be linked to abstract and generic concepts (such as short phrases) that are also visually grounded.
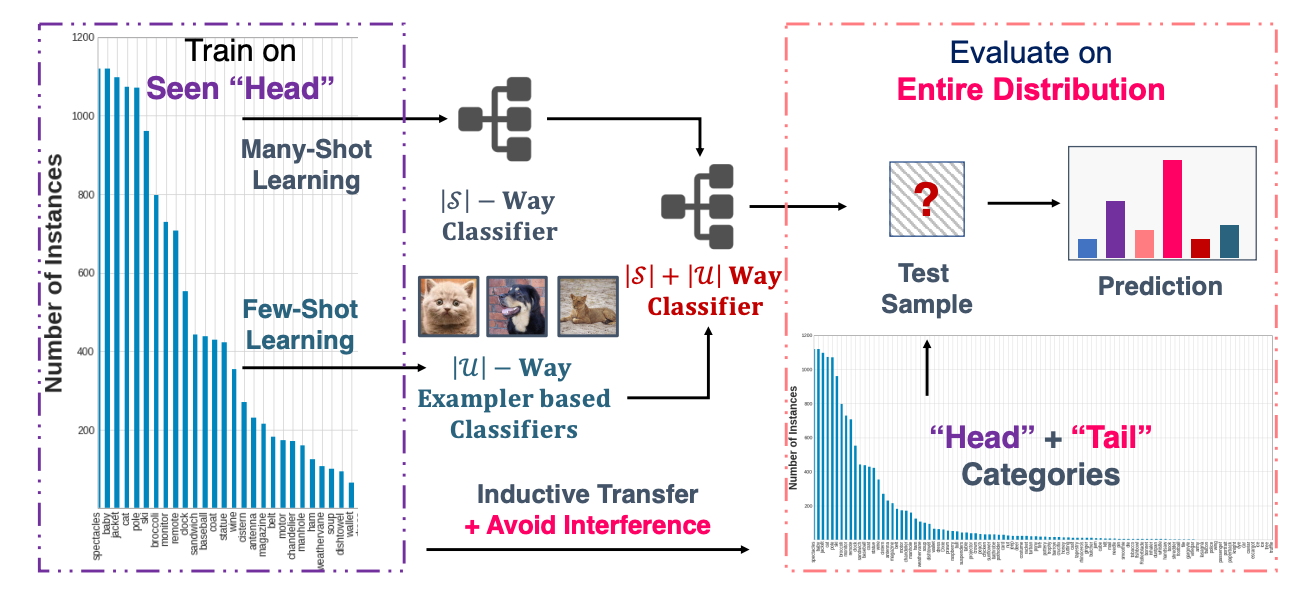
We investigate the problem of generalized few-shot learning (GFSL), using dictionary based classifier synthesis.
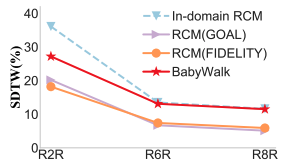
We propose BabyWalk, a novel navigation agent that learns navigate by decomposing long instructions into shorter ones (BabySteps) and completing them sequentially.
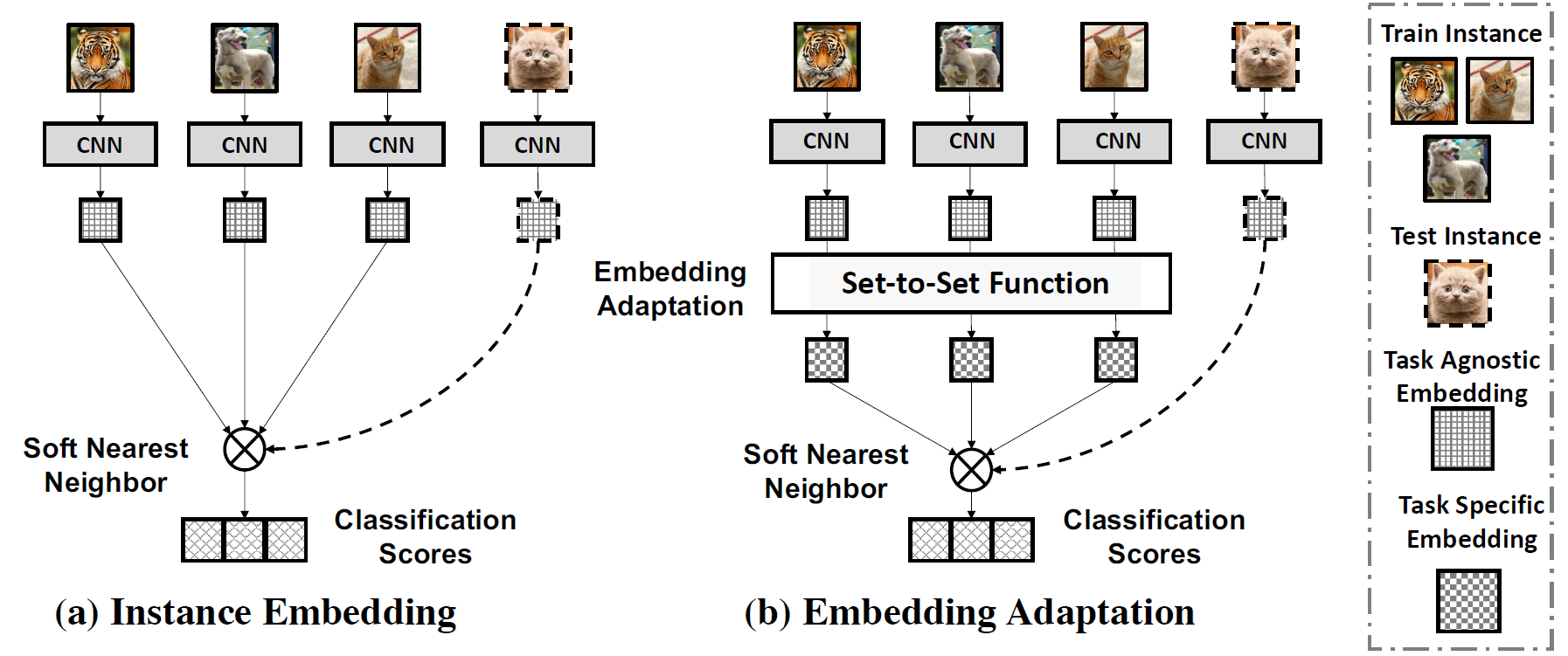
We propose a novel approach to adapt the instance embeddings to the target classification task with a set-to-set function, yielding embeddings that are task-specific and discriminative.
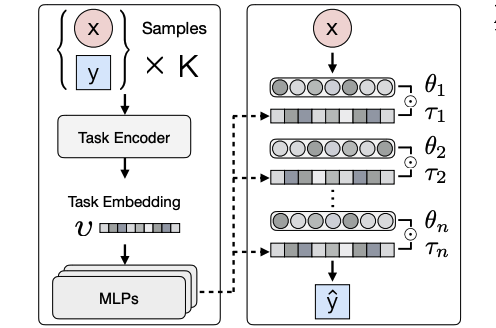
One important limitation of MAML is that they seek a common initialization shared across tasks, which made it suffering from adapting tasks of a multimodal distribution. This paper propose a generic method that augment MAML with the capability of identifying the task mode using a model based learner, such that it can adapt quickly with a few gradient updates.
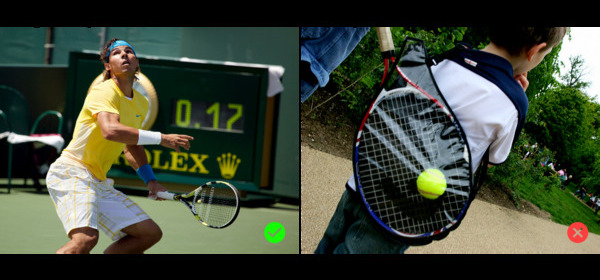
This paper presents an alternative evaluation task for visual-grounding systems: given a caption the system is asked to select the image that best matches the caption from a pair of semantically similar images. The system's accuracy on this Binary Image SelectiON (BISON) task is not only interpretable, but also measures the ability to relate fine-grained text content in the caption to visual content in the images.
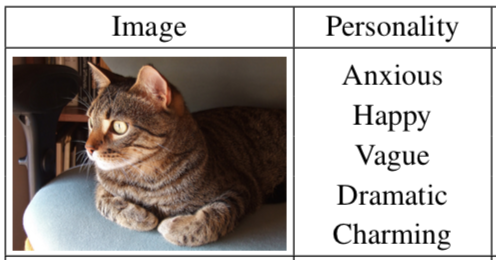
We define a new task, Personality-Captions, where the goal is to be as engaging to humans as possible by incorporating controllable style and personality traits. We collect and release a large dataset of 201,858 of such captions conditioned over 215 possible traits.
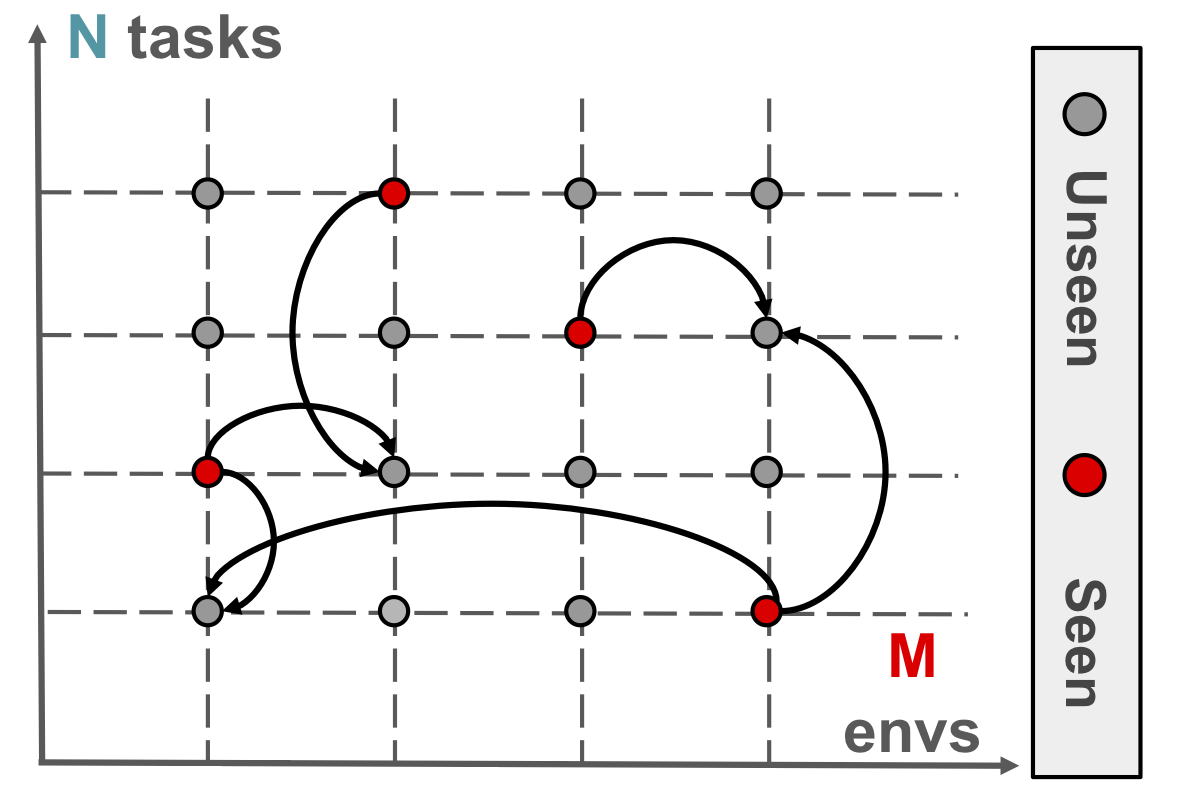
In this paper, we consider the problem of learning to simultaneously transfer across both environments (ENV) and tasks (TASK), probably more importantly, by learning from only sparse (ENV, TASK) pairs out of all possible combinations. We propose a compositional neural network which depicts a meta rule for composing policies from the environment and task embeddings.
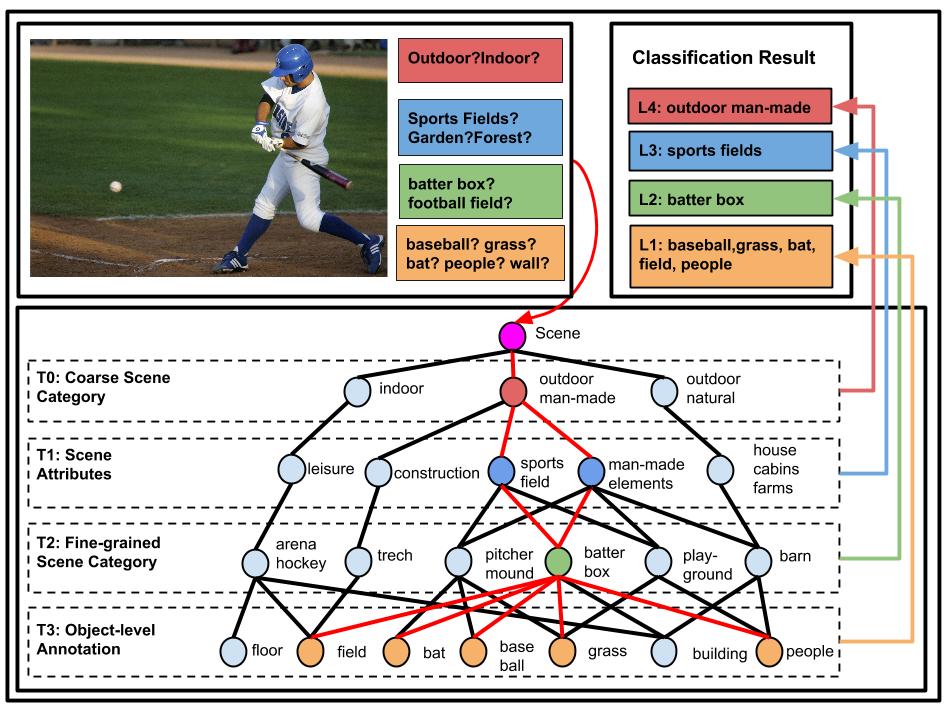
We propose a generic structured model that leverages diverse label relations to improve image classification performance. It employs a novel stacked label prediction neural network, capturing both inter-level and intra-level label semantics. The design of this framework naurally extends to leverage partial observations in the label space to inference the rest label space.
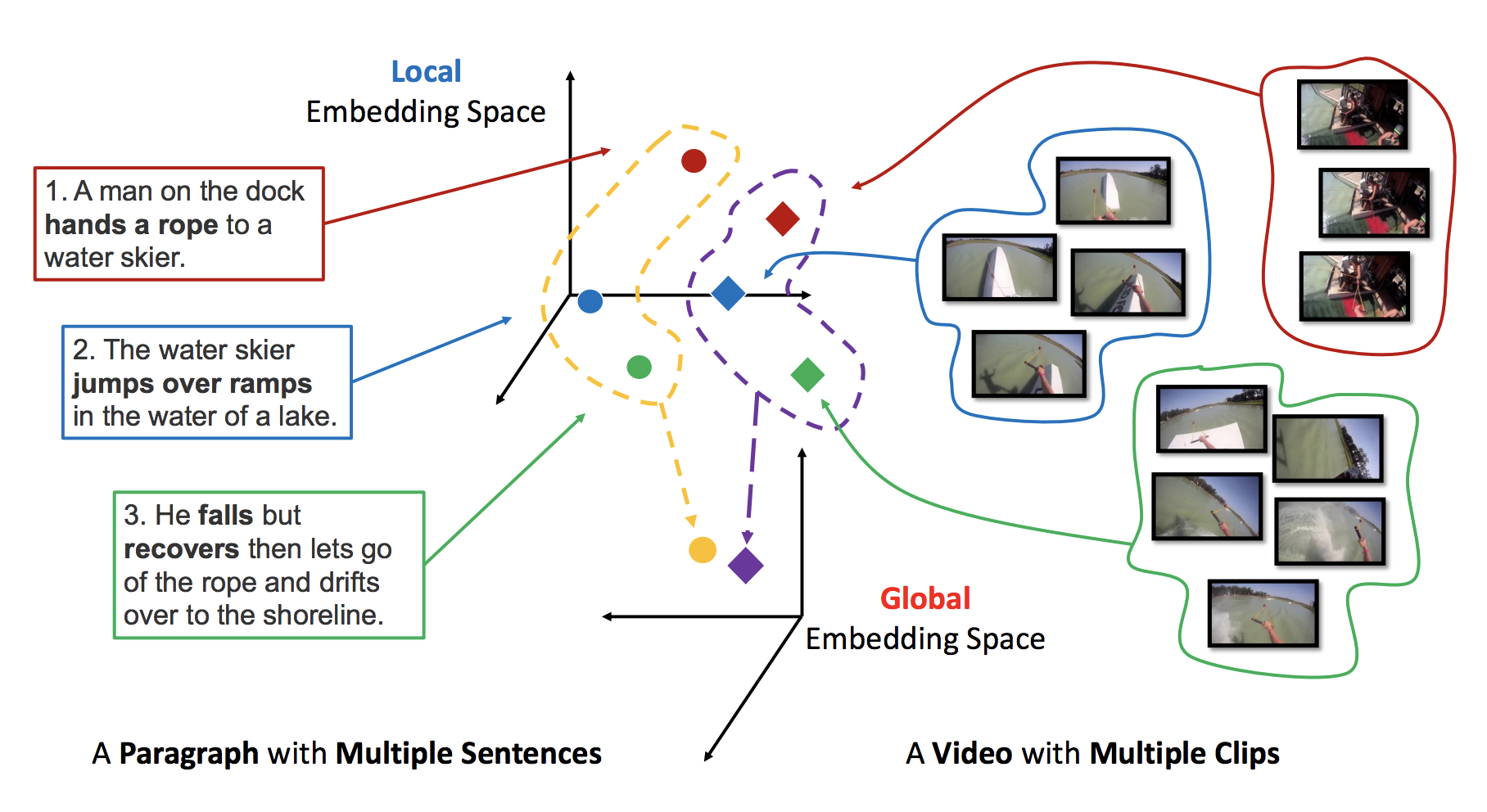
Visual data and text data are composed of information at multiple granularity. In this paper, we investigate the modeling techniques for such hierarchical sequential data where there are correspondences across multiple modalities.
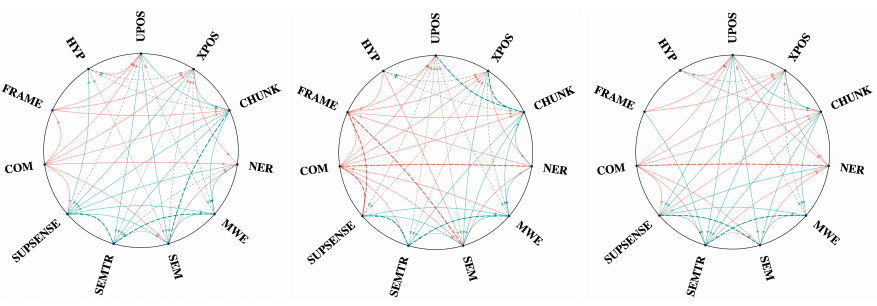
We study three general multi-task learning (MTL) approaches on 11 sequence tagging tasks. Our extensive empirical results show that in about 50\% of cases, jointly learning all 11 tasks improves either learning tasks independently or pairwise learning of tasks. We also show that pairwise MTL can inform us what tasks can benefit others or what tasks can be benefited if they are learned jointly. We additionally identify tasks that can always benefit others as well as tasks that can always be harmed by others.

We show the design of the decoy answers has a significant impact on how and what the learning models learn from the datasets. In particular, the resulting learner can ignore the visual information, the question, or the both while still doing well on the task. Inspired by this, we propose automatic procedures of how to remedy such design deficiencies.
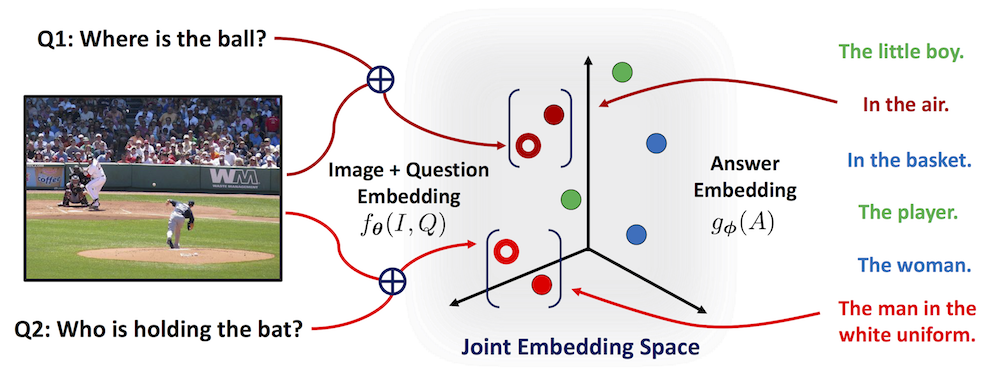
We propose a novel probabilistic model for visual question answering. The key idea is to infer two sets of embeddings: one for the image and the question jointly and the other for the answers. The learning objective is to learn the best parameterization of those embeddings such that the correct answer has higher likelihood among all possible answers.
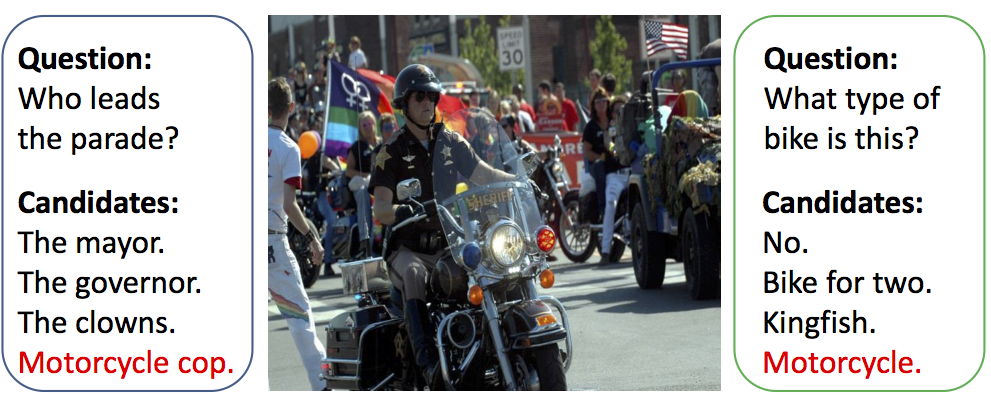
We investigate the problem of cross-dataset adaptation for visual question answering. Our goal is to train a Visual QA model on a source dataset but apply it to another target one. Analogous to domain adaptation for visual recognition, this setting is appealing when the target dataset does not have a sufficient amount of labeled data to learn an "in-domain" model.
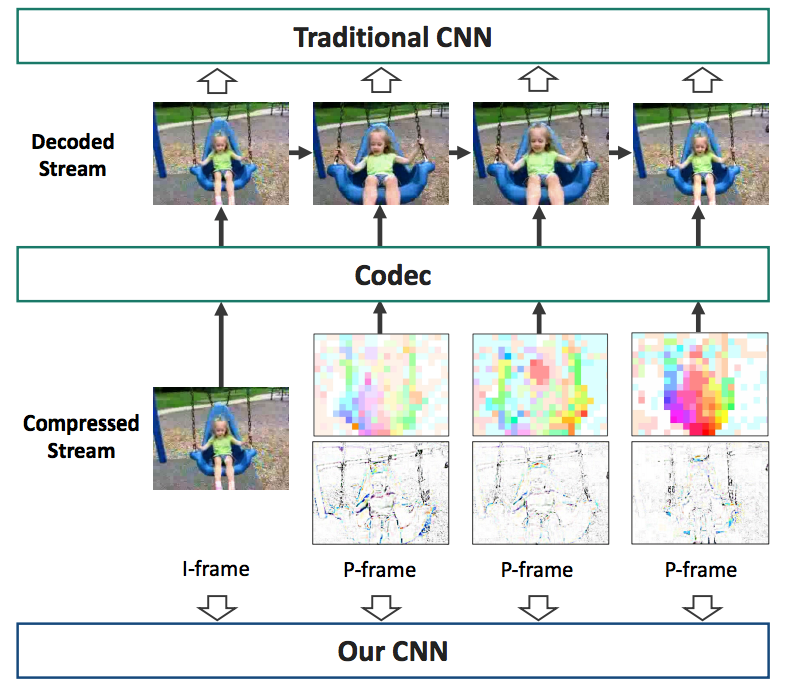
Training robust deep video representations has proven to be much more challenging than learning deep image representations and consequently hampered tasks like video action recognition. Motivated by the fact that the superfluous information can be reduced by up to two orders of magnitude with video compression techniques, in this work, we propose to train a deep network directly on the compressed video, devoid of redundancy

We show the ability of our framework to improve semantic segmentation performance in a variety of settings. We learn models for extracting a holistic LabelBank from visual cues, attributes, and/or textual descriptions. We demonstrate improvements in semantic segmentation accuracy on standard datasets across a range of state-of-the-art segmentation architectures and holistic inference approaches.
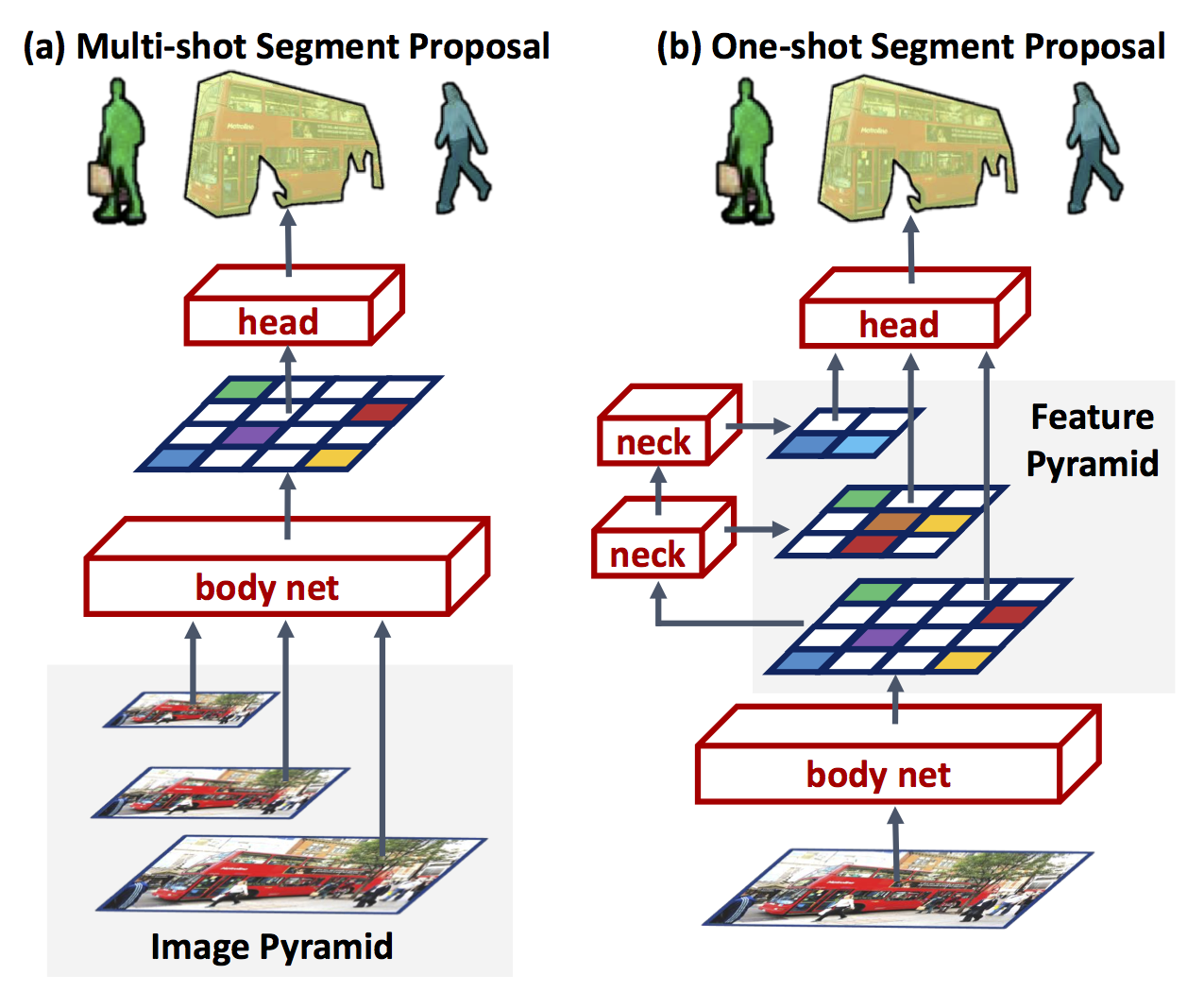
We present a novel segment proposal framework, namely FastMask, which takes advantage of the hierarchical structure in deep convolutional neural network to segment multi-scale objects in one shot. Through leveraging feature pyramid and sliding-window region attention, we made instance proposal not only fast but more accurate.
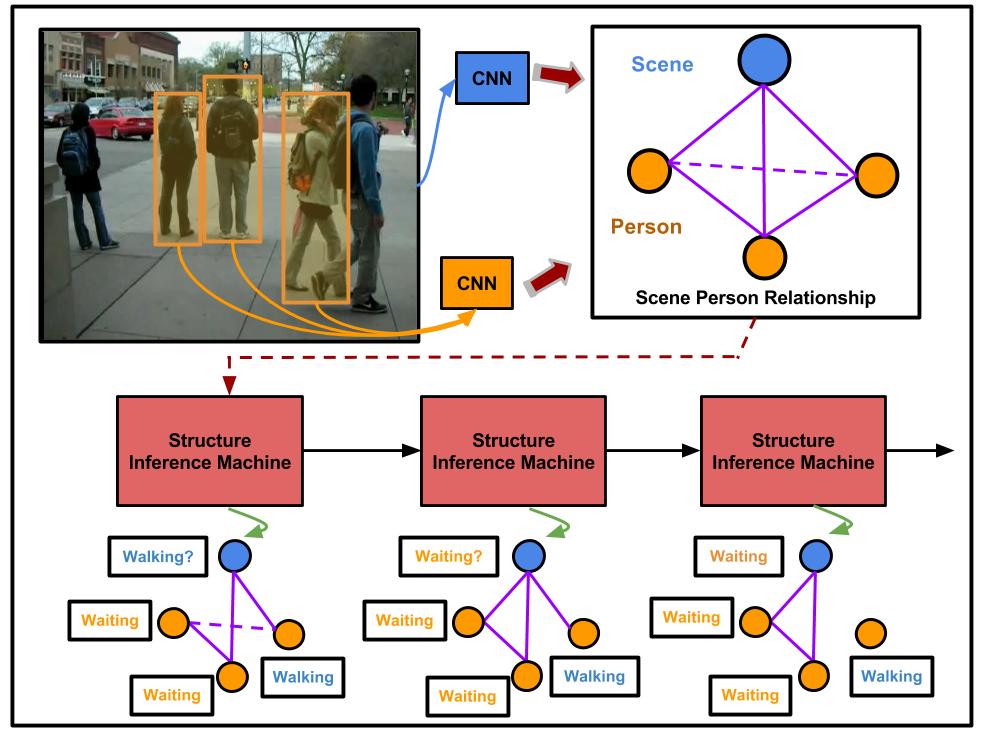
We propose a method to integrate graphical models and deep neural networks into a joint framework with a sequential prediction approximation, modeled by recurrent neural network. This framework simultaneously predicts the underline structure of interactions between people and inferences the corresponding labels for individual and group.