Learning Structured Inference Neural Networks with Label Relations
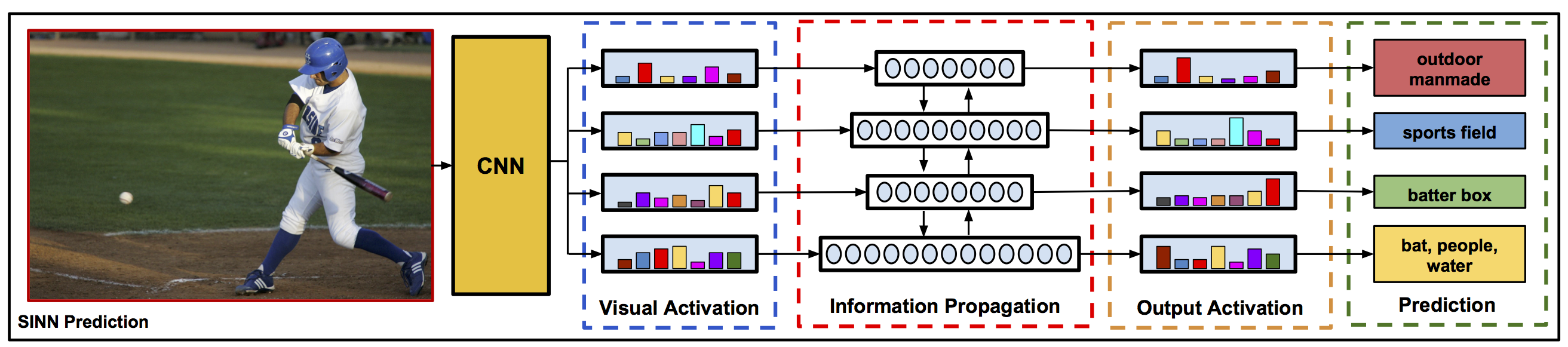
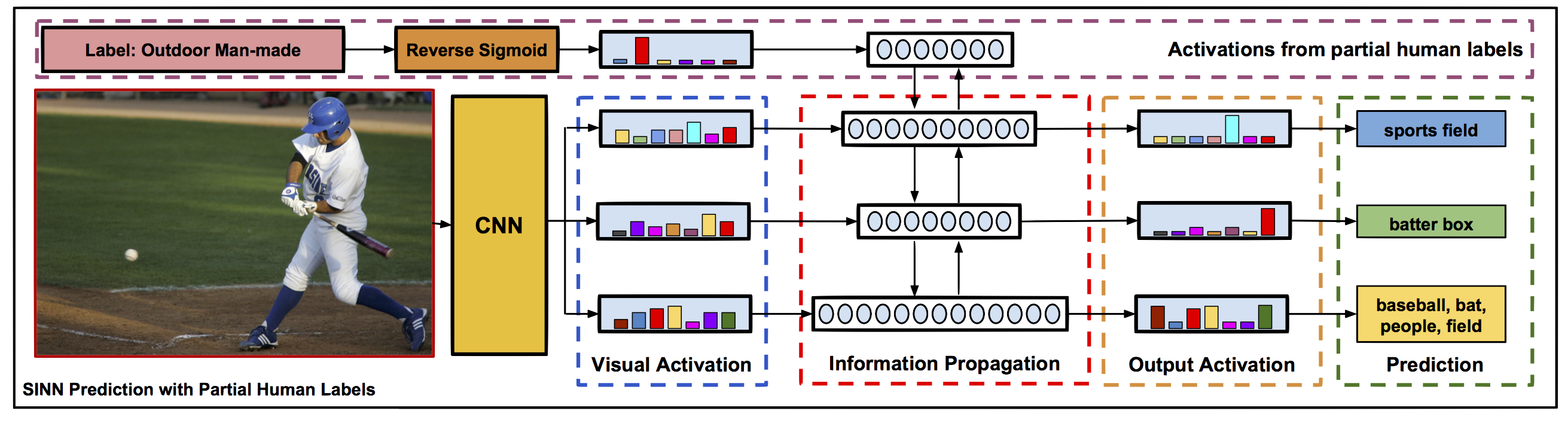
Abstract: Images of scenes have various objects as well as abundant attributes, and diverse levels of visual categorization are possible. A natural image could be assigned with fine-grained labels that describe major components, coarse-grained labels that depict high level abstraction or a set of labels that reveal attributes. Such categorization at different concept layers can be modeled with label graphs encoding label information. In this paper, we exploit this rich information with a state-of-art deep learning framework, and propose a generic structured model that leverages diverse label relations to improve image classification performance. Our approach employs a novel stacked label prediction neural network, capturing both inter-level and intra-level label semantics. We evaluate our method on benchmark image datasets, and empirical results illustrate the efficacy of our model.
